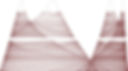
Publications
Dimensionally consistent learning with Buckingham Pi | ||
A concise guide to modelling the physics of embodied intelligence in soft robotics | ||
Enhancing computational fluid dynamics with machine learning | ||
An empirical mean-field model of symmetry-breaking in a turbulent wake | ||
Ensemble-SINDy: Robust sparse model discovery in the low-data, high-noise limit, with active learning and control | ||
Modern Koopman Theory for Dynamical Systems | ||
Kernel learning for robust dynamic mode decomposition: linear and nonlinear disambiguation optimization | ||
Optimal Sensor and Actuator Selection Using Balanced Model Reduction | ||
On the role of nonlinear correlations in reduced-order modelling | ||
PySINDy: A comprehensive Python package for robust
sparse system identification | Alan A. Kaptanoglu, Brian M. de Silva, Urban Fasel, Kadierdan Kaheman, Andy J. Goldschmidt, Jared Callaham, Charles B. Delahunt, Zachary G. Nicolaou, Kathleen Champion, Jean-Christophe Loiseau, J. Nathan Kutz, Steven L. Brunton PySINDy: A comprehensive Python package for robust sparse system identification. Journal of Open Source Software, 7(69), 3994, | |
Applying machine learning to study fluid mechanics | ||
Promoting global stability in data-driven models of quadratic nonlinear dynamics | ||
Sparse nonlinear models of chaotic electroconvection | ||
Data-driven aerospace engineering: Reframing the industry with machine learning. | ||
Nonlinear stochastic modeling with Langevin regression. | ||
Data-driven discovery of Koopman eigenfunctions for control | ||
Data-driven resolvent analysis | ||
Learning dominant physical processes with data-driven balance models | ||
Modeling synchronization in forced turbulent
oscillator flows | ||
Robust Principal Component Analysis for Particle Image Velocimetry | ||
Modal Analysis of Fluid Flows: Applications and Outlook | ||
Machine Learning for Fluid Mechanics | S. L. Brunton, B. R. Noack, and P. Koumoutsakos Annual Review of Fluid Mechanics, 52:477--508, 2020 | |
Data-driven discovery of coordinates and governing equations | ||
Randomized Matrix Decompositions using R | N. B. Erichson, S. Voronin, S. L. Brunton, and J. N. Kutz Journal of Statistical Software , 89(11):1–48 , 2019 | |
A Unified Framework for Sparse Relaxed Regularized Regression: SR3 | P. Zheng, T. Askham, S. L. Brunton, J. N. Kutz, and A. Y. Aravkin IEEE Access, 7(1):1404--1423, 2019 | |
Deep learning for universal linear embeddings of nonlinear dynamics | B. Lusch, J. N. Kutz, S. L. Brunton Nature Communications, 9(1):4950, 2018 | |
Sparse identification of nonlinear dynamics for model predictive control in the low-data limit | E. Kaiser, J. N. Kutz, and S. L. Brunton Proceedings of the Royal Society A, 474(2219), 2018 | |
Neural-inspired sensors enable sparse, efficient classification of spatiotemporal data | T. Mohren, T. L. Daniel, S. L. Brunton, and B. W. Brunton Proceedings of the National Academy of Sciences, 115(42):10564–10569, 2018 | |
Predicting shim gaps in aircraft assembly with machine learning and sparse sensing | K. Manohar, T. Hogan, J. Buttrick, A. G. Banerjee, J. N. Kutz, and S. L. Brunton Journal of Manufacturing Systems, 48(C):87-95, 2018 | |
Data-Driven Sparse Sensor Placement for Reconstruction: Demonstrating the Benefits of Exploiting Known Patterns | K. Manohar, B. W. Brunton, J. N. Kutz, and , S. L. Brunton IEEE Control Systems Magazine, 38(3):63-86, 2018 | |
Sparse reduced-order modeling: Sensor-based dynamics to full-state estimation | J. C. Loiseau, B. R. Noack, and S. L. Brunton Journal of Fluid Mechanics, 844:459–490, 2018 | |
Constrained sparse Galerkin regression | J. C. Loiseau and S. L. Brunton Journal of Fluid Mechanics, 838:42–67, 2018 | |
Modal Analysis of Fluid Flows: An Overview | K. Taira, S. L. Brunton, S. T. M. Dawson, C. W. Rowley, T. Colonius, B. J. McKeon, O. Schmidt, S. Gordeyev, V. Theofilis, and L. S. Ukeiley AIAA Journal, 55(12):4013–4041, 2017 | |
Intracycle angular velocity control of cross-flow turbines | B. Strom, S. L. Brunton, and B. Polagye Nature Energy, 2(17103):1–9, 2017 | |
Chaos as an intermittently forced linear system | S. L. Brunton, B. W. Brunton, J. L. Proctor, E. Kaiser, and J. N. Kutz Nature Communications, 8(19):1–9, 2017 | |
Data-driven discovery of partial differential equations | S. H. Rudy, S. L. Brunton, J. L. Proctor, and J. N. Kutz Science Advances, 3:e1602614, 2017 | |
Sparse sensor placement optimization for classification | B. W. Brunton, S. L. Brunton, J. L. Proctor, and J. N. Kutz SIAM Journal on Applied Mathematics, 76(5):2099–2122, 2016 | |
Discovering governing equations from data: Sparse identification of nonlinear dynamical systems | S. L. Brunton, J. L. Proctor, and J. N. Kutz Proceedings of the National Academy of Sciences, 113(15):3932-3937, 2016 | |
Network Structure of Two-Dimensional Isotropic Turbulence | K. Taira, A. G. Nair, and S. L. Brunton Journal of Fluid Mechanics, 795(R2):1–11, 2016 | |
Finite-time Lyapunov exponents for inertial particles in an unsteady fluidsentations of nonlinear dynamical systems for control | S. Madhavan, S. L. Brunton, and J. J. Riley Physical Review E, 93:033108, 2016 | |
Closed-loop turbulence control: Progress and challenges | S. L. Brunton and B. R. Noack | |
Generalizing dynamic mode decomposition to a larger class of datasets | J. H. Tu, C. W. Rowley, D. M. Luchtenburg, S. L. Brunton, and J. N. Kutz | |
Long-time uncertainty propagation using generalized polynomial chaos and flow map composition | D. M. Luchtenburg, S. L. Brunton, and C. W. Rowley | |
State-space identification of reduced-order unsteady aerodynamic models for feedback control | S. L. Brunton, S. T. M. Dawson, and C. W. Rowley | |
Reduced-order unsteady aerodynamic models at low Reynolds numbers | S. L. Brunton, C. W. Rowley, and D. R. Williams | |
Fast computation of finite-time Lyapunov exponent fields for unsteady flows | S. L. Brunton and C. W. Rowley Chaos 20, 017503, 2010 |